Paper published in IEEE IoT Journal 2021
Pavlopoulou, Niki, and Edward Curry. “IoTSAX: A Dynamic Abstractive Entity Summarisation Approach with Approximation and Embedding-based Reasoning Rules in Publish/Subscribe Systems.” IEEE Internet of Things Journal (2021).
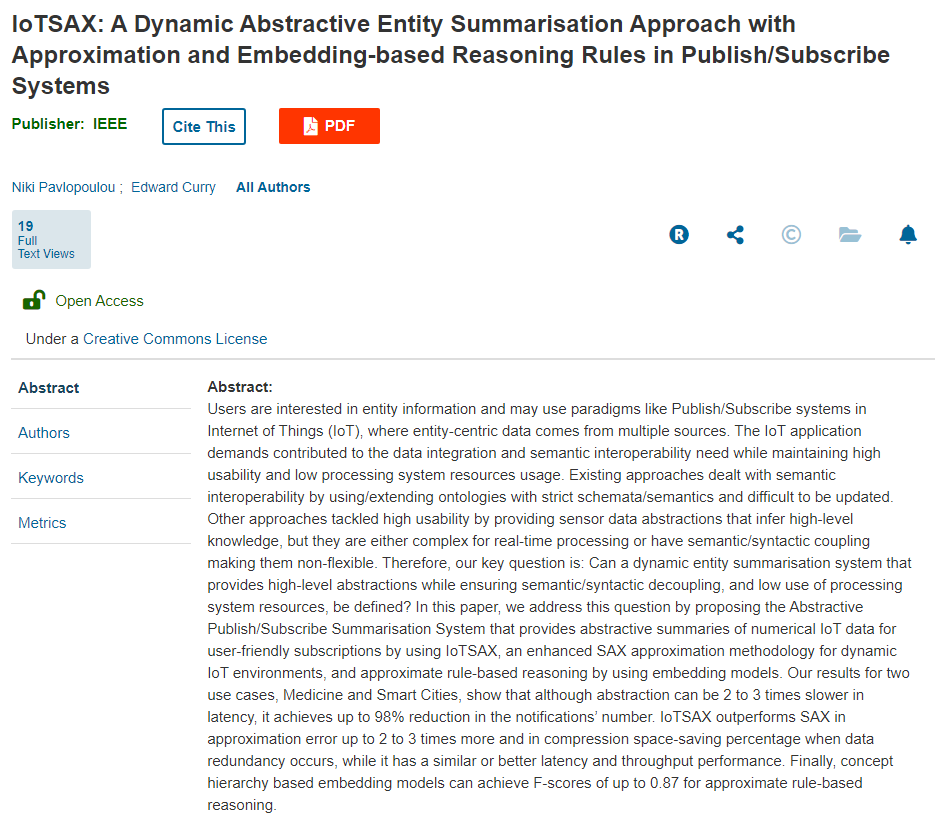
Abstract
Users are interested in entity information and may use paradigms like Publish/Subscribe systems in Internet of Things (IoT), where entity-centric data comes from multiple sources. The IoT application demands contributed to the data integration and semantic interoperability need while maintaining high usability and low processing system resources usage. Existing approaches dealt with semantic interoperability by using/extending ontologies with strict schemata/semantics and difficult to be updated. Other approaches tackled high usability by providing sensor data abstractions that infer high-level knowledge, but they are either complex for real-time processing or have semantic/syntactic coupling making them non-flexible. Therefore, our key question is: Can a dynamic entity summarisation system that provides high-level abstractions while ensuring semantic/syntactic decoupling, and low use of processing system resources, be defined? In this paper, we address this question by proposing the Abstractive Publish/Subscribe Summarisation System that provides abstractive summaries of numerical IoT data for user-friendly subscriptions by using IoTSAX, an enhanced SAX approximation methodology for dynamic IoT environments, and approximate rule-based reasoning by using embedding models. Our results for two use cases, Medicine and Smart Cities, show that although abstraction can be 2 to 3 times slower in latency, it achieves up to 98% reduction in the notifications’ number. IoTSAX outperforms SAX in approximation error up to 2 to 3 times more and in compression space-saving percentage when data redundancy occurs, while it has a similar or better latency and throughput performance. Finally, concept hierarchy based embedding models can achieve F-scores of up to 0.87 for approximate rule-based reasoning.